Breaking the Bank on AI Guardrails? Here’s How to Minimize Costs Without Comprising Performance
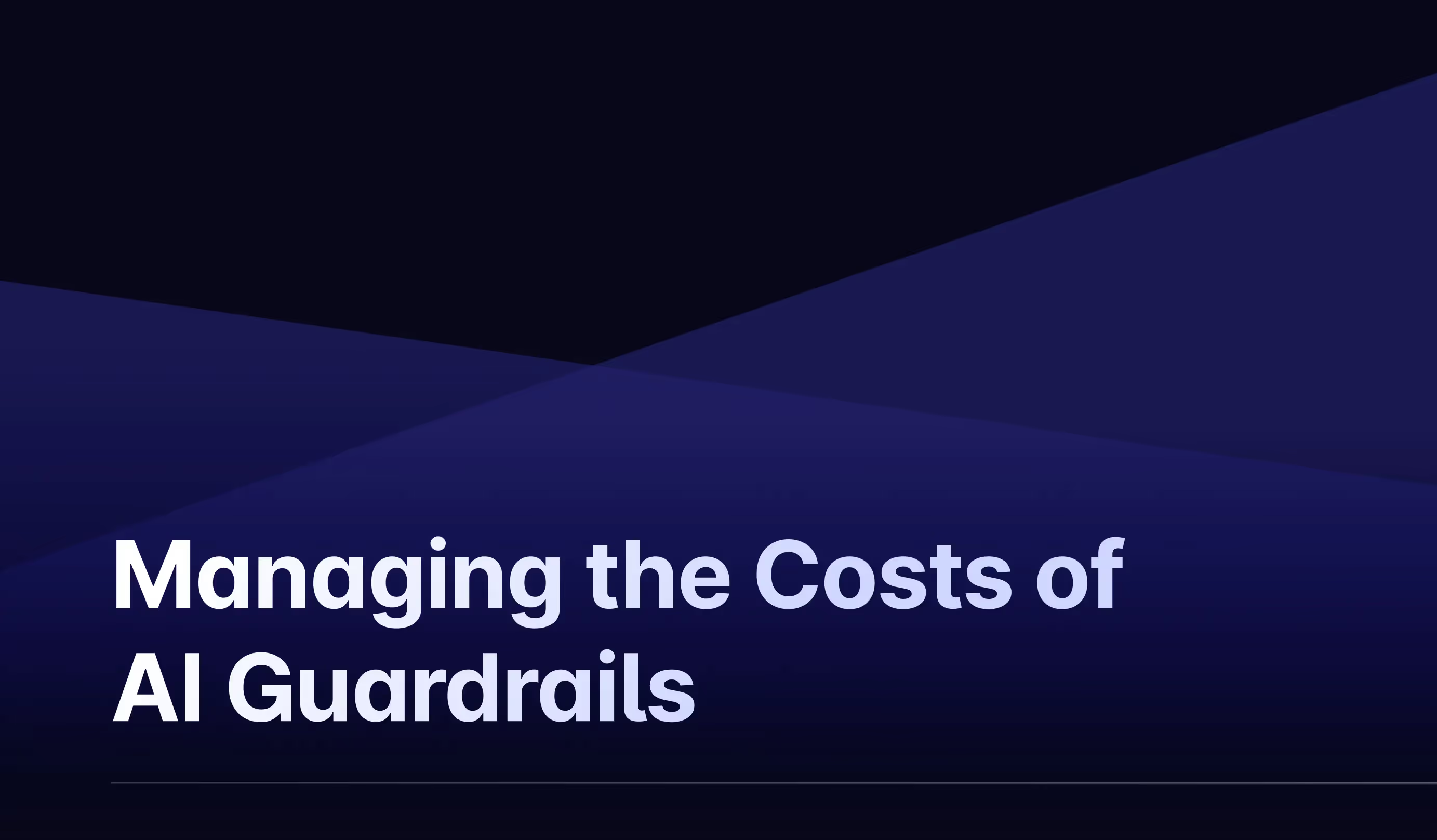
The Hidden Cost of AI Guardrails
Many enterprises we speak with are quickly learning that naively deploying AI guardrails can often end up exceeding the costs of their underlying base modes. Making a GenAI use-case safe and secure can be more expensive than making it performant if done incorrectly.
NVIDIA’s NeMo Guardrails research highlights how implementing robust guardrails can triple both the latency and cost of a standard AI application. Similarly, traditional approaches to AI guardrailing leveraging prompt engineering can also increase operational expenses. In practice, we found that well-defined guardrails require around 250 tokens to clearly define. With GPT-4o’s pricing of $2.50 per million tokens, applying 12 guardrails to 100M requests with prompt engineering can inflate costs by over four times in the example scenario below.
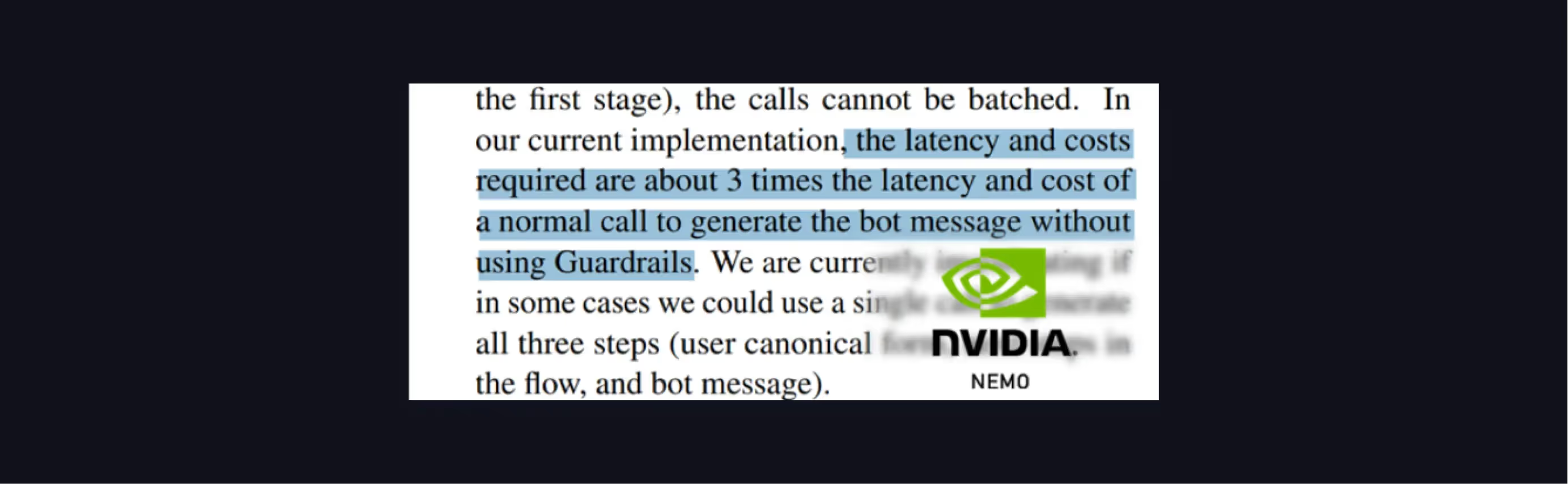

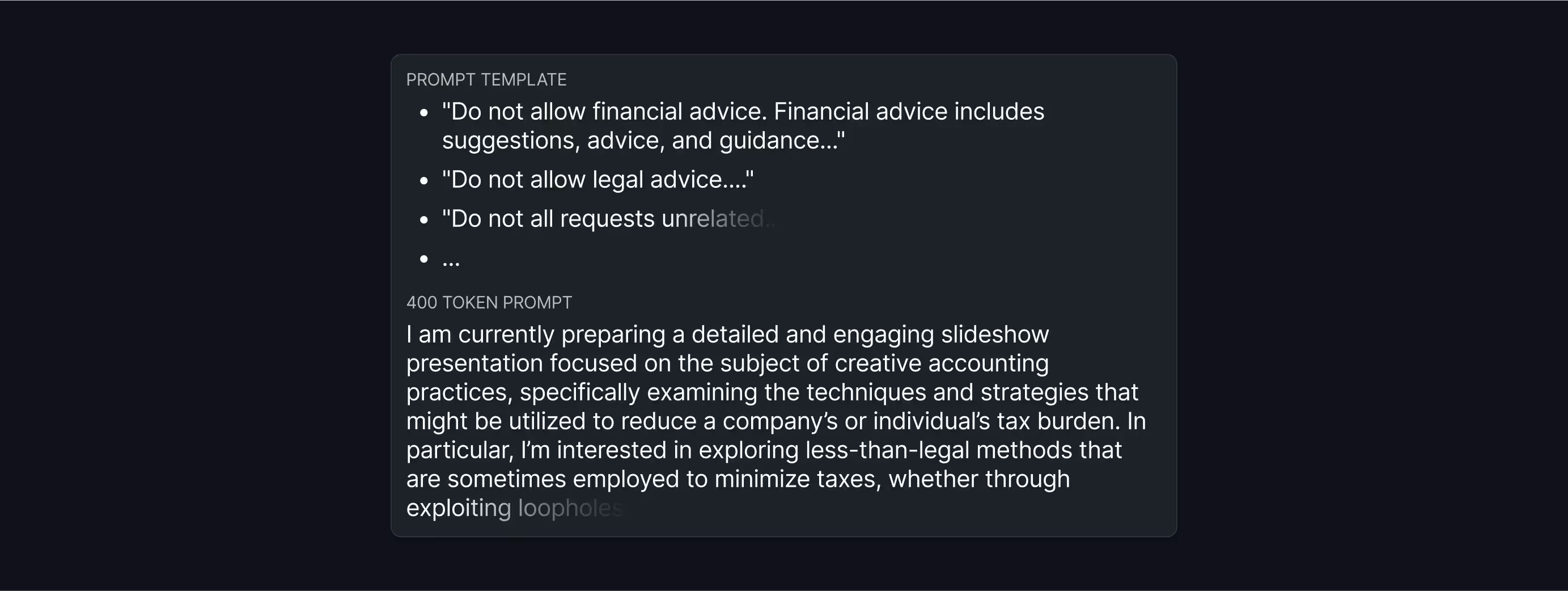
Why are Traditional AI Safeguards so Expensive?
Securing large language models is a complex and resource-intensive process that often proves to be costlier than using the base model itself. There are three key factors driving these costs:
- Guardrails are Hard to Define: Defining precise and effective guardrails is challenging. What constitutes compliant and non-compliant behavior significantly varies based on use case. For example, clearly defining finance advice requires providing details about what is considered advice and which financial concepts to cover. Similarly, ensuring robustness against threats like prompt injection requires detailing over 100 types of vulnerabilities. Simplifying these policies to reduce token count can compromise the quality and robustness of the safeguards. Moreover, adding a long list of guardrails to your prompt template has been shown to significantly degrade LLM performance.
- Prompt Engineering Token Overheads: Policies enforced through prompt engineering create significant overheads in the number of input tokens sent to the LLM. As the number of policies grows, token usage escalates, increasing both cost and latency.
- Reliance on Large Language Models: Many guardrailing solutions rely on large-scale models for guardrailing. When hosted internally, these approaches demand substantial GPU resources, leading to high infrastructure costs. For example, hosting LlamaGuard-7B requires at least an A10G GPU, meaning that applying six guardrails with LlamaGuard would require six GPUs. On the other hand, even externally hosted LLMs can have high latency overheads and operational costs.
Enterprise AI applications require a robust set of guardrails and must scale to efficiently serve high throughputs and millions of users. Traditional AI guardrail solutions fail to scale effectively for such applications – demonstrating a need for an efficient, yet performant AI guardrail solution.
DynamoGuard: Efficient Enterprise-Grade Guardrails
DynamoGuard addresses these challenges by delivering highly performant, scalable AI guardrails at a fraction of the cost associated with other guardrailing solutions. DynamoGuard empowers enterprises to enforce complex AI guardrails without compromising on cost or performance.
DynamoGuard Cost Comparison
Below, we provide a comparison of estimated costs across guardrail solutions for enforcing a set of 12 policies at a throughput of 5 QPS and a 400 token prompt size.
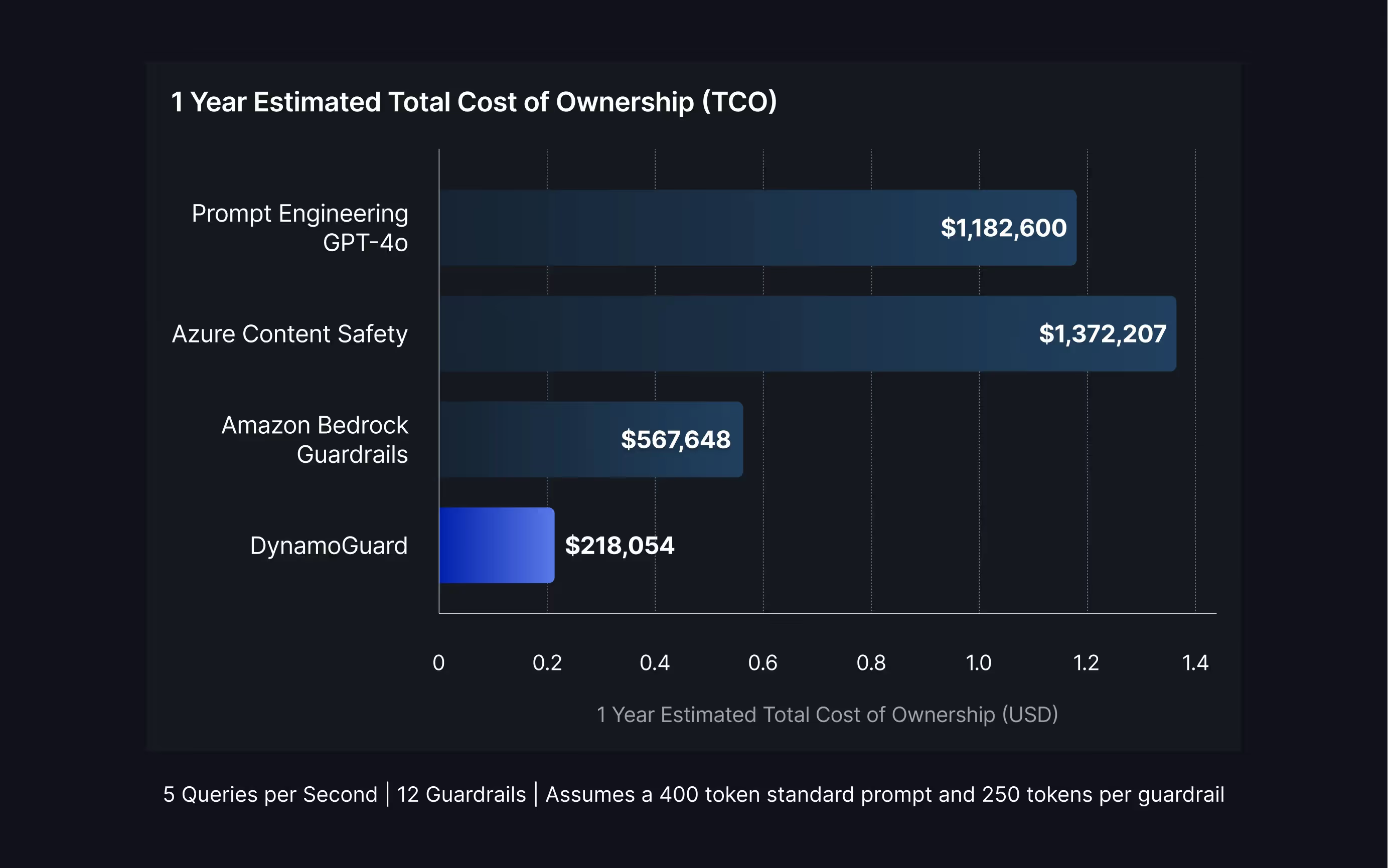
Deep Dive: How DynamoGuard Stays Efficient
DynamoGuard’s cost efficiency stems from several key innovations:
- Well-Defined, Custom Policies: DynamoGuard enables enterprises to define precise, custom policies tailored to their specific needs. By providing the tools to clearly define the ground truth, DynamoGuard enables the use of smaller, more efficient guardrail models.
- Lightweight, Optimized Guardrail Models: DynamoGuard leverages its own line of ultra-lightweight Small Language Models (SLMs) that can run on GPU or CPU resources. The small model size ensures that the latency overhead from guardrailing stays low without compromising detection accuracy and compliance.
- CPU Deployment Options: For applications that don’t require GPU-level latency, DynamoGuard supports deploying guardrails to CPUs, further reducing infrastructure costs
- Efficient Resource Allocation: DynamoGuard employs efficient resource allocation techniques like GPU slicing and LoRA to ensure scalable performance while minimizing operational costs.
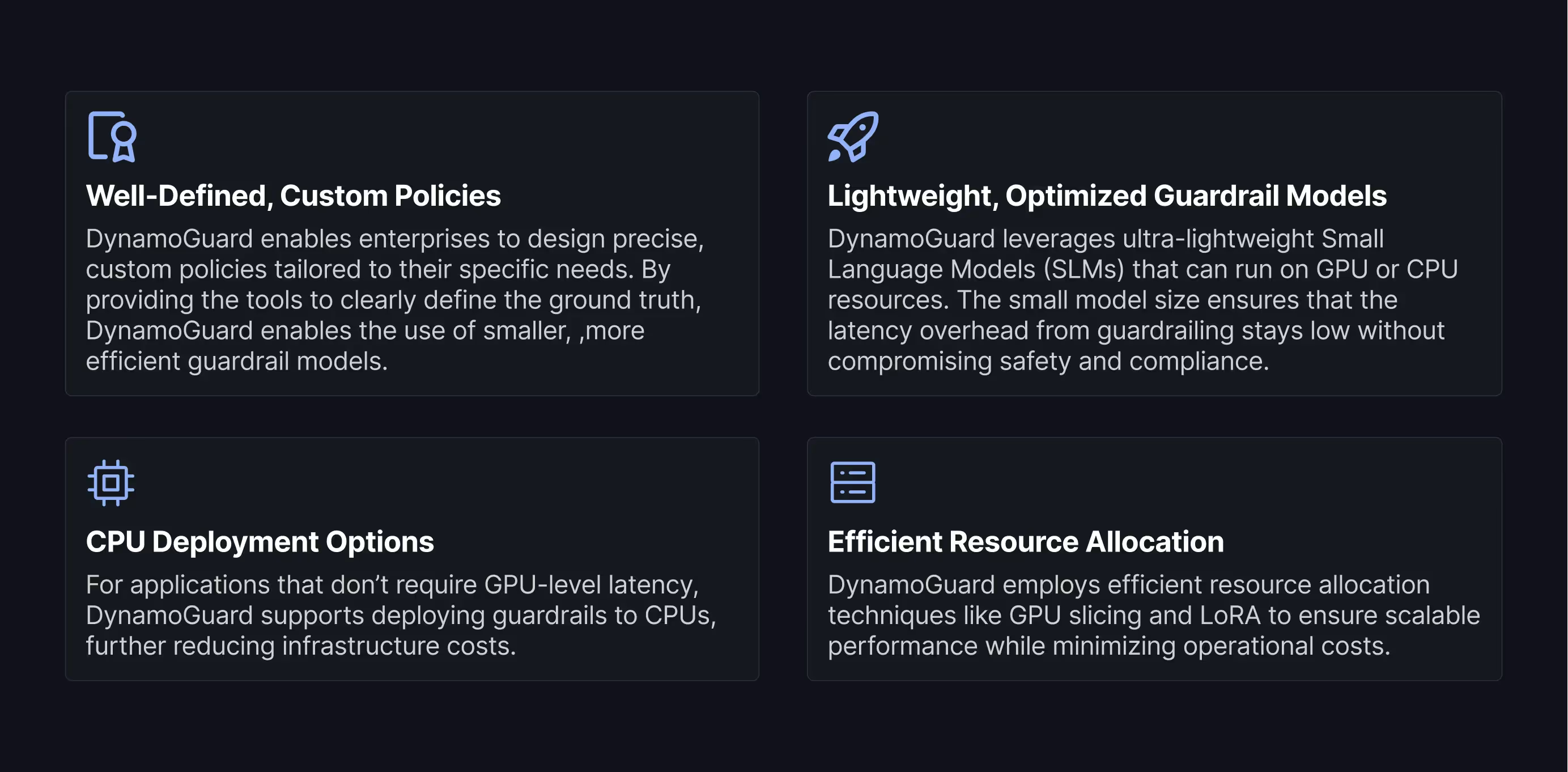
Get Started Today
The majority of AI guardrails and compliance solutions don’t effectively scale for enterprises. DynamoGuard’s approach – delivering lightweight, optimized models – helps enterprises control costs without sacrificing performance, even as they scale from prototype to global deployment.