Dynamo 8B: A Multilingual Foundation for Global Enterprises
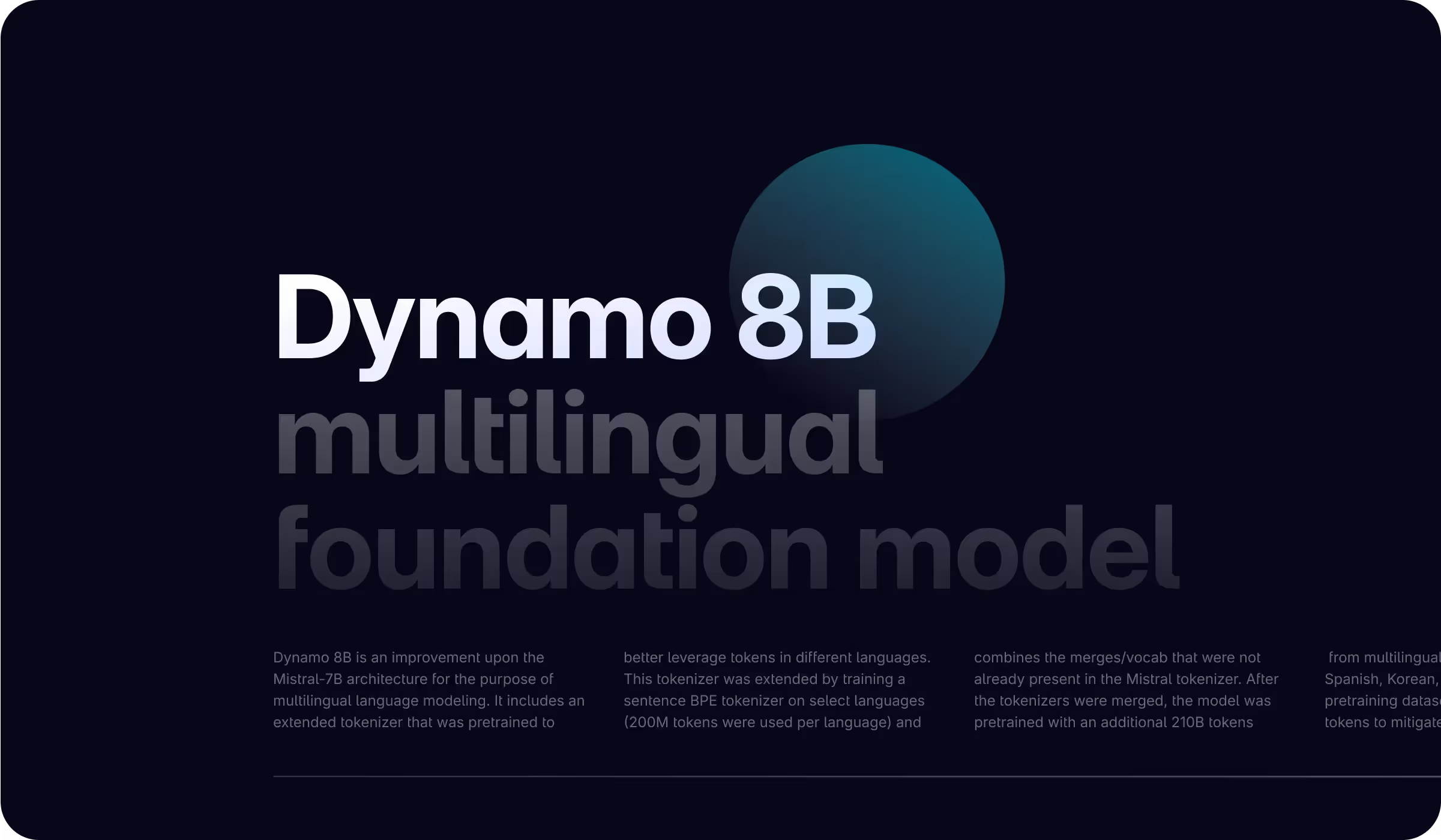
.avif)
Model specifications:
- Supported languages: English, German, Spanish, Korean, Italian, Turkish
- Input token limit: 128k tokens*
- Output token limit: 128k tokens*
- License: At the moment, Dynamo 8B is released under a research-only license.
*Pretraining on the multilingual dataset was done with a sequence length of 4096 tokens
Why we built Dynamo 8B
Even with the constant stream of exciting updates in the LLM space, there is a relative lack of investment in non-English languages, which has resulted in a gap in performance between English and other languages for open-source language models. We built Dynamo 8B to address this gap in multilingual LLM offerings.
We are excited about the downstream applications that Dynamo 8B will support. AI teams today are struggling to address the challenge of unsafe user queries and LLM outputs, which results in major compliance challenges for enterprises that are looking to deploy the technology. As language models like LlamaGuard and Phi-2 were developed to act as more lightweight guardrail models to regulate LLM inputs and outputs, we are excited for Dynamo 8B to similarly enable safe and compliant usage of LLMs globally across a diverse set of languages.
That is why we are releasing Dynamo 8B in connection with the launch of DynamoGuard, a software platform for training guardrail models to enforce custom AI governance policies in enterprise LLMs. We envision leveraging Dynamo 8B to expand DynamoGuard’s capabilities to enable safer and more compliant LLM usage across the globe. Dynamo 8B's ability to achieve leading multilingual performance empowers AI teams to more safely deploy AI systems to their international users, employees, and partners while promoting adherence to emerging AI governance standards.
Training details
Dynamo 8B is an improvement upon the Mistral-7B architecture for the purpose of multilingual language modeling. It includes an extended tokenizer that was pretrained to better leverage tokens in different languages. This tokenizer was extended by training a sentence BPE tokenizer on select languages (200M tokens were used per language) and combines the merges/vocab that were not already present in the Mistral tokenizer. After the tokenizers were merged, the model was pretrained with an additional 210B tokens from multilingual datasets like German, Spanish, Korean, Italian, and Turkish texts. The pretraining dataset also incorporated English tokens to mitigate catastrophic forgetting.
Dynamo 8B has not been instruction fine-tuned and has not undergone alignment using techniques like reinforcement learning from human feedback. The intention behind crafting this model is to provide the research community with a model to explore vital multilingual capabilities that enable widespread use of LLMs globally.
Evaluation details
In our recent evaluation, we used several multilingual benchmarks to assess our model's capabilities. These benchmarks included PAWS, XCOPA, and xstorycloze, all part of EleutherAI's evaluation harness. All of the runs were performed with 32bit precision. Here is an in-depth description of each benchmark we used:
- PAWS (Paraphrase Adversaries from Word Scrambling): This benchmark evaluates the understanding of paraphrasing and language structure by distinguishing between semantically equivalent and inequivalent pairs of sentences. In a multilingual context, it challenges the model to perform this task across different languages, testing its capability to understand nuanced meaning beyond mere word-to-word translation.
- XCOPA (Cross-lingual Choice of Plausible Alternatives): Originally developed as COPA (Choice of Plausible Alternatives) for English, this benchmark assesses causal reasoning abilities in a model. XCOPA extends this to multiple languages. It presents the model with a premise and two alternatives, where the model must identify the most plausible cause or effect of the given premise, requiring deep understanding of the context and the logical relationships.
- XSTORYCLOZE: An extension of the Story Cloze Test, this benchmark completes stories after prompting with just the first few sentences. In a multilingual setting, this test evaluates the model's narrative understanding and its ability to predict logical and coherent story endings in different languages.
.avif)
Reach out to learn more
Our released Dynamo 8B model is available on HuggingFace here.
To learn how to leverage Dynamo 8B for your AI solutions, schedule your free demo.